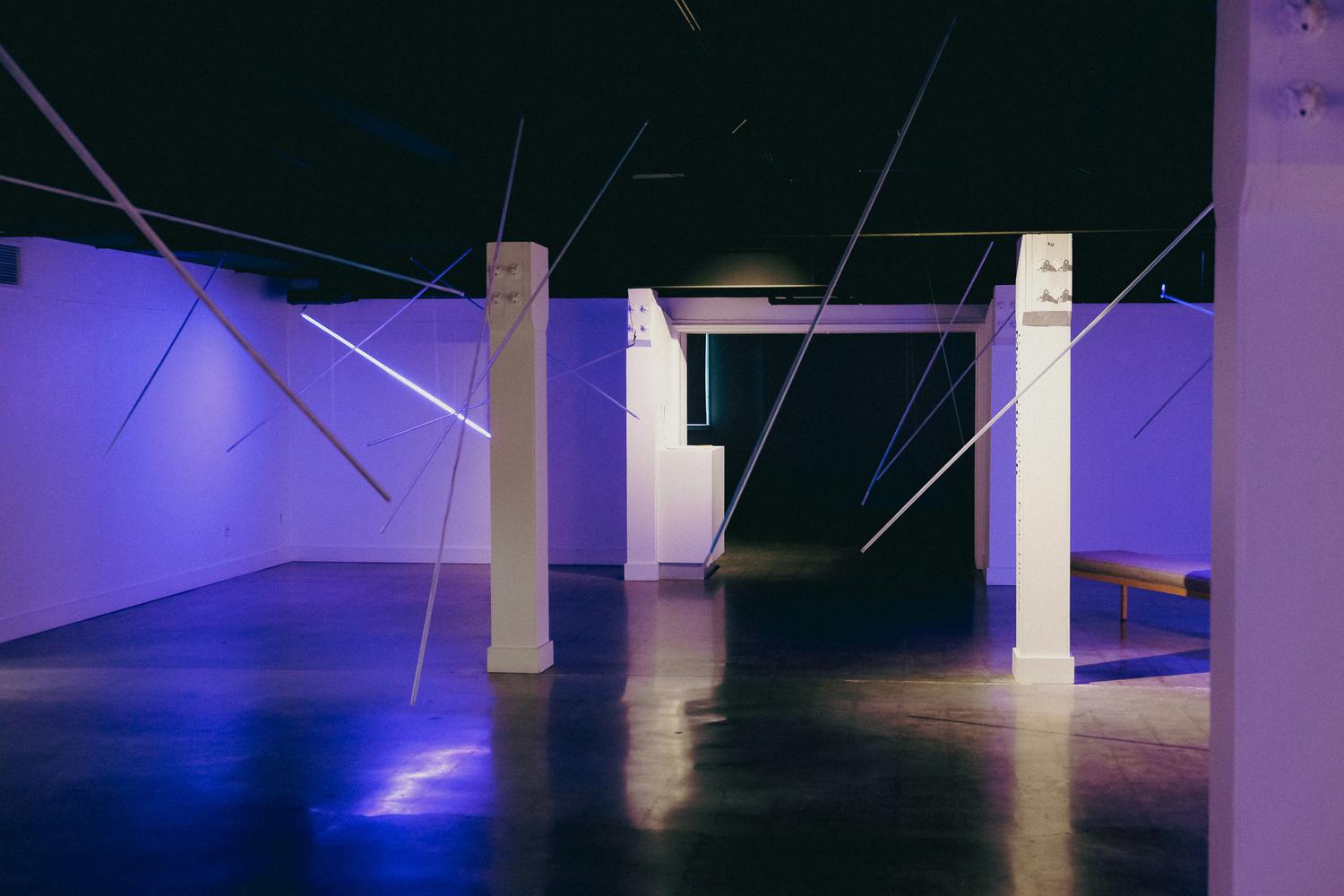
By Ari Lauer-Frey
Artificial Intelligence (AI) is here, and it is here to stay. AI tools have been implemented in countless sectors of modern life, from finance to healthcare to education. And, as indicated by the Trump administration’s announcement of the Stargate project on Jan. 21, the advancement of AI will be continuous and rapid. Led by OpenAI in partnership with other significant AI actors, the project represents a major move in AI infrastructure, with $100 billion already invested in the construction of data centers and a total of $500 billion planned to be allocated for such development.
OpenAI has become dominant in the world of AI resources due to ChatGPT, a highly skilled generative AI-trained chatbot, as well as their image generation services — providing answers for users from questions about the migrational patterns of sea birds to what Cookie Monster would look like if he had an obsession with celery instead. More recently, OpenAI has received attention and criticism for their new 4o image generation tool, an advancement of their imaging abilities with loosened rules around image creation, including the capability to quite accurately recreate distinct art styles. This new feature led to an excessive production of images in the style of Hayao Miyazaki’s Studio Ghibli and was even used by the White House in a controversial post on X (formerly Twitter) depicting a notable U.S. Immigration and Customs Enforcement arrest in the Studio Ghibli art style. Miyazaki’s past comment on a 2016 AI animation project by Japanese media company, Dwango, utilizing his signature style, asserting it to be “an insult to life itself,” exemplifies the increasing tensions and overlaps between AI and artists.
AI’s increasing presence is a concern shared among many artists, such as studio arts major Bey Anderson (‘25): “It does feel like a big deal to me; it’s becoming such a big part of daily life now, and I’m just not a fan of all the AI in our lives. It feels unnerving — every time I’m on Instagram, every reel is some crazy AI ‘art’ stuff, and I just don’t like it.” This moment raises important questions about the current and continuing influence AI tools have upon the art world — shaping the ways we create and the ways we approach, value and understand artistic practices.
Though tools like 4o may feel quite new, artificial and automated intelligence used for artistic creation has existed within the larger context of digital art, consisting of endless variations in the relationship between artists and technology since the 1960s. AI art has arguably existed since 1972, with artist Harold Cohen’s creation of AARON, a series of computer programs that could independently create artworks using robotic drawing and painting tools. Additionally, though many associate the term as synonymous with generative AI, generative art has existed since computer graphic software, with artists finding opportunities in the realm of coding.
Professor Mare Hirsch, whose class “Art from Code” focuses on such practices, explains generative art as being an approach focused on the relationship between input and output: “In kind of its purest sense, I think generative art is something where you have some system of rules, and the output to that could potentially be different every single time. So you kind of have this aspect of endless variation, unpredictability, noise.” This input-output structure reflects a possible difference between generative art and other traditions (like painting) in that the role of the artist is largely one of translator, with artistic intention being found in the shaping of the framework or tool through which translation takes place.
The approach of generative artists, in some ways, has been the basis for today’s widely used AI Image generation tools such as DALL-E, Stable Diffusion and Canva’s Magic Media instrument. Such tools are trained on data lakes, often comprising hundreds of millions of images and their related text descriptions, helping the models learn the correlation between key terms and visual elements with great accuracy.
However, in regards to human participation, these tools present a significant change: there is little to no individual agency in the creation of a framework, and, as in the case of OpenAI’s services, the input of a prompt is often the extent of human creativity. As these models that diminish human participation increase in popularity, the dynamics between artists and AI may trend toward competition rather than collaboration. As creative writing major and artist Megan Riehle (‘25) explains, “There is an urge to get scared and feel like the work that we do as contemporary artists will not be valued or appreciated or circulated in the same ways that it has been in the past because there’s this other presence that overshadows it.” While AI may not affect all arts or artists to the same extent, this concern is legitimated in AI’s effect on vocational artists. New research published by Harvard Business Review found that “Within a year of introducing image-generating AI tools, demand for graphic design and 3D modeling freelancers decreased by 17.01%.”
Notably, there are various concerns about AI art beyond its effects on the labor market. Image generation is the most energy-consumptive use of generative AI, with a study by Hugging Face and Carnegie Mellon University finding that “Generating 1,000 images with a powerful AI model, such as Stable Diffusion XL, is responsible for roughly as much carbon dioxide as driving the equivalent of 4.1 miles in an average gasoline-powered car.” The process of training these models has also brought up ethical concerns regarding intellectual property, as many of them have trained with content indiscriminately taken from the internet, leading to various copyright lawsuits.
Historically, digital art has presented an interesting challenge to the ideas of ownership and intellectual property that AI is now further complicating. Digital art — particularly during the information revolution of the ’90s-2000s — has often offered an alternative to the investment-based model of the art market by reshaping notions of distribution and ownership. As Hirsch explains, “So much of computational art and the history of code art has had this really strong and kind of fierce culture of open source, which means, basically, anyone can borrow this code, they can make their own version of it.” Importantly, though, these communities of open-source attitude have been opt-in environments. In the case of the aforementioned lawsuits, such AI companies are making immense profits off of significant amounts of work from artists who have not signed off on their use or, more frequently, are not aware of it. And yet there are others who see AI as a generally positive force of disruption, such as Marxist AI artist and Tiktoker @leessyndikaat, who asserts that “Intellectual property is a bourgeois right” and “protecting these class interests is counter-revolutionary and Liberal, and I’m not really interested in protecting that.”
Despite all of these reasons to be concerned, there are still ways in which AI art does not (and may never) match up to the value of human artists. Though AI-created artworks have passed the Turing Test — a test of a machine’s ability to exhibit human-like intelligence — in being graded in comparable visual quality to human artworks, studies — such as one published by the Association for Computing Machinery — have shown that knowledge that a work is AI-produced negatively impacts its qualitative assessment. While this judgment might be explained as a momentary bias against such technology, it seems more likely to be indicative of where and how we find value in art. Perhaps the most obvious is the value we place upon the creative process, the great work that an artist puts into making something that feels meaningful. “I think the creative process is, in a lot of ways, all that matters. The process of making something, the act of creation itself, is beautiful and complete,” affirms Riehle. And the creative process is absent from AI — or at least the parts of the creative process that are most important.
As Professor Justin Tiehen argues in his article “Existential risk and value misalignment,” one of the primary factors that limit AI from being comparable to human intelligence, a goal hypothesized in the idea of AGI (artificial general intelligence), is its inability to make existential choices. Unlike AI, we humans have the ability to make decisions that contradict our core/final values. With this capability for value misalignment, we are able to make existential choices — choices that fundamentally change our core/final values. Whereas generative AI will always be making some form of derivation based on its consumed data and fixed goal, humans harness an unpredictability that is inherent to the innovation, novelty and magic of the creative process. And while AI will undoubtedly change and complicate human life, perhaps it can also make us more aware of the importance of art, creativity and the many other things that make us distinctly human.